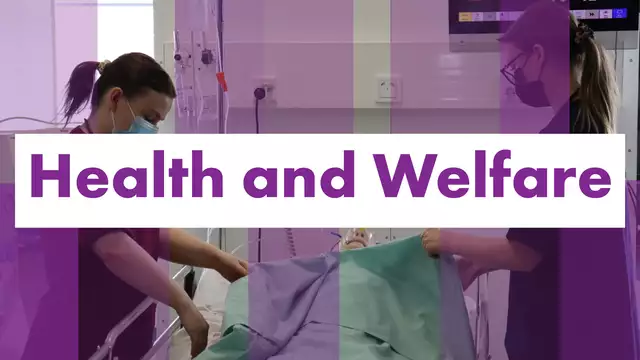
Leonardo Espinosa-Leal, PhD, Senior Lecturer in Big Data Analytics. Arcada University of Applied Sciences. E-mail: leonardo.espinosaleal
Artificial intelligence has become the modern paradigm in almost all areas of knowledge. Significant advances in fields like deep neural networks (Goodfellow, 2016) have created algorithms able to rival humans in areas as never before, including vision (LeCun, 1995), language (Greff, 2016), and many others. Nowadays, machines are capable the defeating the human masters on almost any board game (Silver, 2018).
Performing tasks at the human level mean that somehow human can be replaced or repurposed in less repetitive tasks. Ignoring philosophical or sociological discussions about how this technological revolution can impact, positively or negatively, the human population in the near or far future in general, it is clear that one of the goals of these advances is the creation of fully autonomous and intelligent embodied agents. For a more general discussion, I encourage the reader to explore recent reference works on the subject (Volti, 2004; Kleinman, 2014)
The advances in robotics made by companies such as Boston Dynamics or SoftBank Robotics seem to bring the ancient dreams of creating artificial humanoids into reality. The secret sauce of these humanoid machines’ success is, apart from the advances in models, hardware, or software, done by highly skilled technical and theoretical experts, the endless homunculus amount of data generated by simple digital users.
Yes, you muggle! In most cases, the human’s digital footprint has been responsible for creating and tagging data (sometimes on purpose, in others as a side subproduct of our web surfing). Data that have helped train these human-level deep learning algorithms. And here is where the problem arises. Powerful tech companies have expanded their services and products created with inherited inequalities within that data.
The digital gap among different societies has allowed the creation of biased datasets. Modern estimations argue that more than half the global population has access to the internet; however, studies have shown that digital skills and access vary by region and gender. For instance, a 2019 study showed that 55% of men used the internet in the USA while only 48% of women did so. Moreover, Only 44% of the population in the developing world and 20% of the people in the least developed world currently have internet access, in contrast to developed regions where over 85% of people have access. Similar inequalities can be found in other areas, such as age group, education level, and socioeconomic demographic information (Statista, n.d.; Pew 2019).
The landmark moment in the history of deep learning is the ImageNet Large Scale Visual Recognition Challenge (ILSVRC) of 2012, won by Alex Krizhevsky (Krizhevsky, 2012). Here, GPU-powered Neural Networks enter the research sphere. ImageNet was an international contest where several research groups competed by bringing their best computer vision algorithms trying to reach the lowest classification error. ImageNet consists of 14,197,122 images organized into 21,841 subcategories. This dataset was compiled by Fei-Fei Li’s group at Stanford (Deng, 2009). This huge dataset has been the reference and the ground truth for new computer vision developments; however, it has been acknowledged recently that it contains flows and biases (Yang, 2020).
Other specific fields use a limited number of standard datasets, for instance, in Indoor Scene Recognition (MIT Indoor Scenes or Stanford 3D Indoor Scene Dataset); Face recognition (WIDER Face or IMDb-Face); Autonomous driving (Waymo Open Dataset or Virtual KITTI ). A quick inspection will tell us how western-urban-male-centric biased are these datasets. I encourage the reader to check the site https://paperswithcode.com/dataset External link, just filter by language to see how English is the dominant language by far, compared to the second in the list.
It is acknowledged that big tech players: GAMMAs (Google, Amazon, Meta (Facebook), Microsoft, Apple) or BATXs (Baidu, Alibaba, Tencent, and Xiaomi) are, with some academic institutions, the primary source of datasets for training artificial intelligence algorithms. These companies overlap in different digital markets and become active competitors in products and services in the digital world. A quick look at the origin of these giant digital behemoths shows implicitly that, in terms of language, English and Chinese are their main interests. Unfortunately, with its diversity of languages, Europe lags behind in developing technological products, exposing its citizens to a new linguistic cybercolonialism.
Natural Language Processing (NLP) is a subfield of linguistics, computer science, and artificial intelligence concerned with the interactions between computers and human language, mainly how to program computers to process and analyze large amounts of natural language data. The goal is a computer capable of “understanding” the contents of documents, including the language’s contextual nuances (Wikipedia, 2019). NLP is expected to become an essential player for improving the experience in Human-Robot Interaction (HRI). In the future, robotic assistants are expected to replace specific human labor or tasks. The success in the interaction between humans and robotic assistants is linked to the inclusion of populations not covered by products with technological limitations in language.
A local case
Finland is a small country in terms of population. Finnish is the primary language globally; only around 5.4 million Finnish-speaking natives are located mainly in the nordic areas (Kotimaisten kielten keskus, 2019). Different academic institutions have made enormous efforts to develop several NLP products in the local Finnish language (Virtanen, 2019; Hämäläinen, 2021). The second official language of Finland is a regional variant of Swedish. Finland has approximately 296,000 Swedish speakers. About 9 million people speak Swedish as their first language (Kotimaisten kielten keskus, 2019). Due to closeness with Sweden, the primary candidate for creating services are the tools developed using the Swedish language from Sweden (Malmsten, 2020). Although inside the Finnish Swedish community, there are identified four regions where the Finnish Swedish dialects are spoken (Ostrobothnia, the autonomous island province of Åland, Åboland, and Nyland (Uusimaa)), from these, there are ten identified dialects (Kotimaisten kielten keskus, n.d.).
Development and study of Finnish Swedish population within Human-Robot Interaction real is a necessary step for developing more inclusive products and services. For instance, a successful campaign named donate your speech was launched in 2020, supported by the Finnish Broadcasting Company (YLE), to encourage Finnish speakers to create a large dataset for training speech recognition algorithms in Finnish (Lahjoitapuhetta, n.d.). Similar initiatives funded by Svenska Kulturfonden have been launched recently, including the MäRI and TaFiDiaAI initiatives led by Arcada and Experience Lab that aim specifically to study and develop products for HRI within the Finnish Swedish speaking population in a Healthcare setup. TaFiDiaAI has been the first initiative for collecting specifically Finnish Swedish dialects (see http://snacka.fi/ External link). More recently, Yle Svenska, supported by Svenska literature, has launched a similar initiative at a significant scale for collecting speech data (www.kielipankki.fi External link, n.d.)
These initiatives, as mentioned earlier, are the first step for the inclusion of minorities within the Finnish society, with a particular focus on healthcare services. There are a lot of challenges ahead, but digitalization and automatization are unavoidable; however, we must agree that for an ethical and inclusive future, we need to consider from the beginning the creation of products and services that include all populations from scratch. In conjunction with the digital industries, researchers and academia must join synergies to build a more inclusive society where AI benefits all its citizens.
Acknowledgments
The author acknowledges the economic support of Svenska Kulturfonden (The Swedish Cultural Foundation in Finland).
Bibliography
Deng, J., Dong, W., Socher, R., Li, L.J., Li, K. and Fei-Fei, L., 2009, June. Imagenet: A large-scale hierarchical image database. In 2009 IEEE conference on computer vision and pattern recognition (pp. 248-255). IEEE.
Goodfellow, I., Bengio, Y. and Courville, A., 2016. Deep learning. MIT press.
GGreff, K., Srivastava, R.K., Koutník, J., Steunebrink, B.R. and Schmidhuber, J., 2016. LSTM: A search space odyssey. IEEE transactions on neural networks and learning systems, 28(10), pp.2222-2232.
Hämäläinen, M., Alnajjar, K., Partanen, N. and Rueter, J., 2021. Finnish Dialect Identification: The Effect of Audio and Text. arXiv preprint arXiv:2111.03800.
www.kielipankki.fi External link. (n.d.). The Swedish version of the Donate Speech campaign has started online | Kielipankki. [online] Available at: https://www.kielipankki.fi/news/the-swedish-version-of-the-donate-speech-campaign-has-started-online/ External link [Accessed 14 Feb. 2022].
Kleinman, D.L. and Moore, K. eds., 2014. Routledge handbook of science, technology and society. London: Routledge.
Kotimaisten kielten keskus. (2019). Languages of Finland – Institute for the Languages of Finland. [online] Available at: https://www.kotus.fi/en/on_language/languages_of_finland External link.
Kotimaisten kielten keskus. (n.d.). Swedish dialects in Finland – Institute for the Languages of Finland. [online] Available at: https://www.kotus.fi/en/on_language/dialects/swedish_dialects_in_finland_7542 External link [Accessed 14 Feb. 2022].
Krizhevsky, A., Sutskever, I. and Hinton, G.E., 2012. Imagenet classification with deep convolutional neural networks. Advances in neural information processing systems, 25.
Lahjoitapuhetta.fi. (n.d.). Lahjoita puhetta. [online] Available at: https://lahjoitapuhetta.fi/ External link [Accessed 14 Feb. 2022].
LeCun, Y. and Bengio, Y., 1995. Convolutional networks for images, speech, and time series. The handbook of brain theory and neural networks, 3361(10), p.1995..
Malmsten, M., Börjeson, L. and Haffenden, C., 2020. Playing with Words at the National Library of Sweden–Making a Swedish BERT. arXiv preprint arXiv:2007.01658.
Pew Research Center (2019). Internet/Broadband Fact Sheet. [online] Pew Research Center: Internet, Science & Tech. Available at: https://www.pewresearch.org/internet/fact-sheet/internet-broadband/ External link.
Silver, D., Hubert, T., Schrittwieser, J., Antonoglou, I., Lai, M., Guez, A., Lanctot, M., Sifre, L., Kumaran, D., Graepel, T. and Lillicrap, T., 2018. A general reinforcement learning algorithm that masters chess, shogi, and Go through self-play. Science, 362(6419), pp.1140-1144.
Statista. (n.d.). Global internet usage rate by gender and market 2019. [online] Available at: https://www.statista.com/statistics/333871/gender-distribution-of-internet-users-worldwide/ External link.
Volti, R., 2005. Society and technological change. Macmillan.
Virtanen, A., Kanerva, J., Ilo, R., Luoma, J., Luotolahti, J., Salakoski, T., Ginter, F. and Pyysalo, S., 2019. Multilingual is not enough: BERT for Finnish. arXiv preprint arXiv:1912.07076.
Wikipedia Contributors (2019). Natural language processing. [online] Wikipedia. Available at: https://en.wikipedia.org/wiki/Natural_language_processing External link.
Yang, K., Qinami, K., Fei-Fei, L., Deng, J. and Russakovsky, O., 2020, January. Towards fairer datasets: Filtering and balancing the distribution of the people subtree in the imagenet hierarchy. In Proceedings of the 2020 Conference on Fairness, Accountability, and Transparency (pp. 547-558).